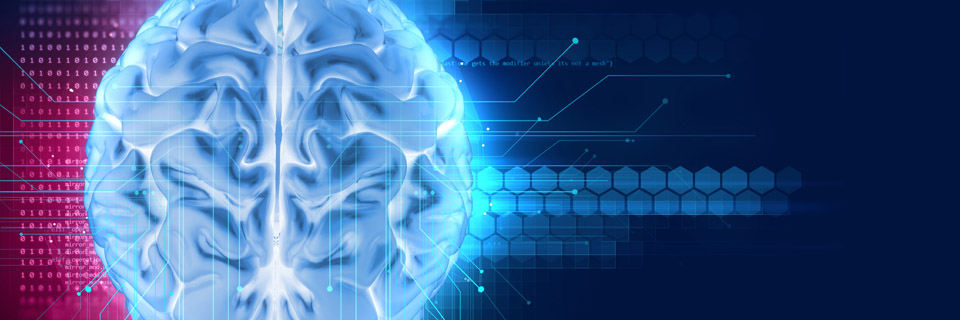
Correlating threat data – how APTs changed cybersecurity
In January 2010, the sedate world of computer security suddenly collided head on with the cyber era that was about to change it forever. In a public statement, Google revealed that during 2009 it had been on the receiving end of a sophisticated Chinese-based cyberattack designed to steal sensitive information from its servers.
TweetSecurity experts were astonished by the admission as well as the event itself. The dam of denial broken, the same attackers were soon connected to incursions against dozens of other US companies. Cyberattacks were nothing new but Aurora seemed to have been going on a vast scale, over a period of years and with a level of success that shocked the industry. Security companies soon coined a new term to explain the type of attack, the Advanced Persistent Threat or APT.
The techniques employed by APTs had been routine for years but the innovation was to use them together. These included long-term reconnaissance probing for weaknesses, installing software backdoors for remote access Trojans (RATs), exploiting software vulnerabilities, stealing user credentials and identities, and exploiting privilege escalation. Most significant of all, if any piece of this infrastructure was discovered, they would adapt, retool and, rewrite malware, and then simply return in a technique dubbed ‘persistence’.
The persistence bit was important because it implied that such attacks weren’t speculative and short-term but part of a long-term strategy built on an industrial scale. Attackers using APTs would keep coming back, over and over, until they found a weakness that let them in.
Defending against this sort of threat was not going to be easy but the security came up with an answer of sorts in the form of better threat analytics harnessed to more sophisticated correlation. The idea was a simple adaptation to the way APTs worked. If attackers tried to get into networks using a coordinated series of tools, malware and manual incursions, defenders needed to see each of these events as part of a larger picture. Finding a single piece of malware or a compromised credential told you something had happened but understanding an attack in situ depended on linking this to other apparently unconnected events elsewhere in the network.
From anomaly to correlation
Anomalies are the basis of all security: a ‘normal’ state is defined by policy and then deviations from that set of an alert. But in modern IT environments, there is no single event that can be described as anomalous so much as he accumulation of these in a pattern.
Finding that pattern is the job of correlation although what is correlated will vary from network to network. Instead of looking for a malicious program or action based on some kind of pre-defined signature or known pattern, correlation is about spotting events inside a network and risk-scoring them using one of two assessments. The first is statistical, through which a chain of causality can be revealed between apparently unconnected events based on mathematical likelihood. This can struggle where events are separated by longer periods of time which is why a second approach, machine learning (sometimes bravely called ‘AI’), has started to attract attention. Critics of machine learning will often point out the way that vendors have taken to using it as a buzz-phrase that overlooks the importance of not swamping defenders with false alarms. If the point of machine learning and AI is to remove the load from human beings and make possible rapid response, loading human decision makers with too much of the wrong data becomes counter-productive.
Equally, it is clear that the layering of security through multiple event monitoring systems can’t indefinitely be managed by humans in real time without simply tying up more and more IT staff. From this perspective, it makes perfect sense that machine-driven attacks must be countered with machine-driven defences that can, in time, detect even new types of incursion based on the ability to rapidly join the dots. For now, this remains a long-term objective that will take years to play out.
Correlation platforms
All major security vendors support some form of correlation and anomaly detection often built around that company’s historical specialisms. These integrate heterogeneous data and alert sources into one global view that focuses on trying to understand how events might be changing over time.
This can be security’s equivalent of ‘nailing jelly to the wall’. Sources of data that feed into an assessment will include user behavior, account activity, events within the layer of privilege elevation (e.g. acquiring the right to access a server or install an application), the appearance of new application, open ports or processes and isolated malware detections.
Any one of these events can be relatively minor on its own, but together can constitute a threat if they are connected. Differentiating one from the other will often be about context, for example an admin accessing an important server at an unusual time of day. Most of the time, this would count as normal but if it happens at the end of a chain of events in which an unknown executable was detected in a separate system, might raise a red flag.
The question is where does a threat detection analysis engine step in and either automatically block an action or require a human operator to intervene to check its legitimacy.
Analytics is not a panacea
A major challenge remains marrying the theory of automated analytics with the reality of where vulnerabilities lie in any network. The problem with correlating simple anomalies (or patterns of anomalies|), is that many attacks don’t need them to gain a foothold. A lot of cyberattacks exploit stolen credentials that are not protected with any additional authentication, in which case what is done with such accounts might look perfect normal: the action of the attacker mimics what the authorised user would do but the defenders have no way of distinguishing one from the other.
Similarly, endpoints are another popular target because they are often permissive zones. From a single PC, users can range around large parts of some networks, reaching out to servers, databases and even cloud assets. Compromising this can be as simple as engineering a user to open a single attachment. Will security systems notice this? On the PC itself, possibly but if not an attacker will almost certainly have found a way in because, if not detected, that action looks perfectly normal in that environment.
The answer to this is to reduce the agency of the PC inside networks and build a trust layer around users. No user, resource, application or connection should ever be granted absolute trust.
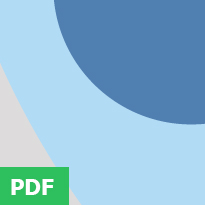
The Forrester Wave™: Privileged Identity Management, Q3 2016
The 10 Providers That Matter Most And How They Stack Up
DOWNLOAD HERE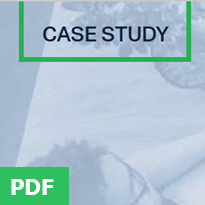
RWE Supply & Trading Secures Against User and Asset-Based Risks
BEYONDTRUST CUSTOMER SUCCESS STORY
DOWNLOAD HERE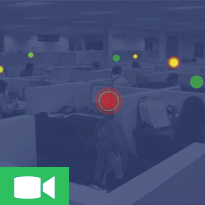
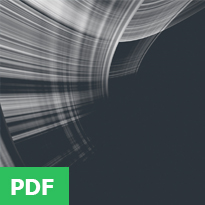